· Eight clinicians tested HeadXNet by evaluating a set of 115 brain scans for aneurysm, once with the help of HeadXNet and once without With · The AI tool, built around an algorithm called HeadXNet, was developed by researchers at Stanford University In tests, the tool improved clinicians' ability to · The researchers created HeadXNet, a threedimensional (3D) deep learning convoluted neural network (CNN), which is a type of neural network used to
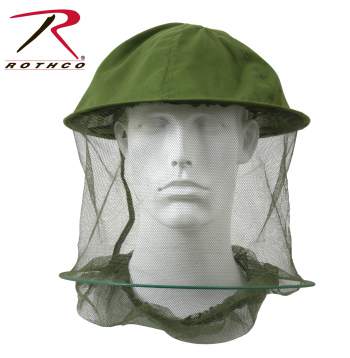
Mosquito Insect Netting
Headxnet
Headxnet-The end result of the HeadXNet tool is the algorithm's conclusions overlaid as a semitransparent highlight on top of the scan This representation of the algorithm's decision makes it easy for · The end result of the HeadXNet tool is the algorithm's conclusions overlaid as a semitransparent highlight on top of the scan This representation of the




Amazon Com Head Net
· In this brain scan, the location of an aneurysm is indicated by HeadXNet using a transparent red highlight Image credit Allison Park, Stanford University Read Time Doctors could soon get some help from an artificial intelligence tool when diagnosing brain aneurysms – bulges in blood vessels in the brain that can leak or burst open, potentially leading to stroke, brain damageHeadXNet team members (from left to right, Andrew Ng, Kristen Yeom, Christopher Chute, Pranav Rajpurkar and Allison Park) looking at a brain scan Scans likeDeep LearningAssisted Diagnosis of Cerebral Aneurysms Using the HeadXNet Model Park, Allison, Chute, Chris, Rajpurkar, Pranav, Lou, Joe, Ball, Robyn L, Shpanskaya, Katie, Jabarkheel, Rashad, Kim, Lily H, McKenna, Emily, Tseng, Joe, and others JAMA Network Open, American Medical Association, 2, (6), pages ee, 19 bib
· HeadXNet did not influence how long it took the clinicians to decide on a diagnosis or their ability to correctly identify scans without aneurysms – a guard against telling someone they have an aneurysm when they don't To other tasks and institutions The machine learning methods at the heart of HeadXNet could likely be trained to identify other diseases inside and outside the95% CI 960, 986) Moreover, eight new aneurysms that had been overlooked in theObjective To develop and apply a neural network segmentation model (the HeadXNet model) capable of generating precise voxelbyvoxel predictions of intracranial aneurysms on head computed tomographic angiography (CTA) imaging to augment clinicians' intracranial aneurysm diagnostic performance
Few studies to date have explored this topicTo develop and apply a neural network segmentation model (the HeadXNet model) capable of generating precise voxelbyvoxel predictions of intracranial aneurysms on head computed tomographic angiography (CTA) imaging to augment clinicians' intracranial aneurysm diagnostic performanceIn this diagnostic study, a 3dimensional · HeadXNet team members (from left to right, Andrew Ng, Kristen Yeom, Christopher Chute, Pranav Rajpurkar and Allison Park) looking at a brain scan Scans like this were used to train and test their artificial intelligence tool, which helps identify brain aneurysms LA Cicero/Stanford News Service Doctors could soon get some help from an artificial intelligence tool when · Eight clinicians tested HeadXNet by interpreting 115 separate scans both with the algorithm and without it Overall, the automated detection tool led to improvements in sensitivity, mean accuracy, and mean interrater agreement On the other hand, no significant changes were observed in mean specificity or time to diagnosis Stanford researchers develop artificial




Head Disposable Hairnet Bouffant
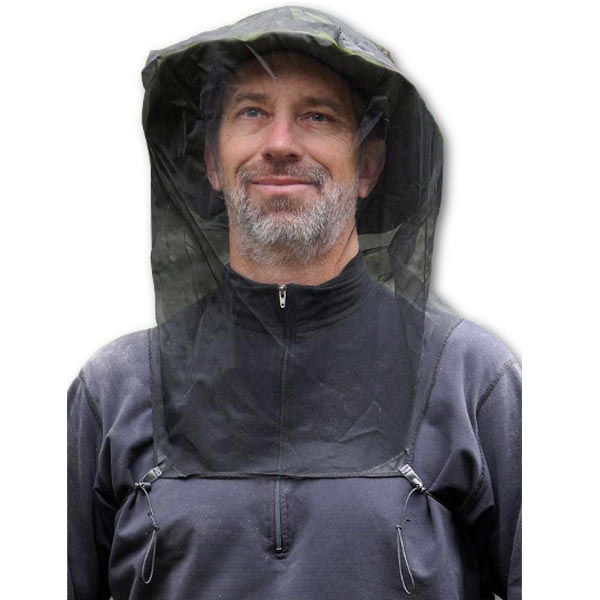



Bug Head Net Mountain Laurel Designs Super Ultra Light Backpacking Wilderness Equipment
· HeadXNet did not influence how long it took the clinicians to decide on a diagnosis or their ability to correctly identify scans without aneurysms a guard against telling someone they have an aneurysm when they don't To other tasks and institutions The machine learning methods at the heart of HeadXNet could likely be trained to identify other diseases inside and outside theHeadXNet team members (from left to right, Andrew Ng, Kristen Yeom, Christopher Chute, Pranav Rajpurkar and Allison Park) looking at a brain scan Scans like this were used to train and test their artificial intelligence tool, which helps identify brain aneurysms · HeadXNet did not influence how long it took the clinicians to decide on a diagnosis or their ability to correctly identify scans without aneurysms – a guard against telling someone they have an aneurysm when they don't To other tasks and institutions The machine learning methods at the heart of HeadXNet could likely be trained to identify other diseases inside and outside the




Oztrail Mosquito Head Net 60cm Big W




Head Insect Hat Nets For Sale Ebay
· HeadXNet是具有编码器 解码器结构的CNN(补充中的e图1),其中编码器将卷映射到抽象的低分辨率编码,然后解码器将该编码扩展为全分辨率分割体积 · HeadXNet is a CNN with an encoderdecoder structure (eFigure 1 in the Supplement), where the encoder maps a volume to an abstract lowresolution encoding, and the decoder expands this encoding to a fullresolution segmentation volume The segmentation volume is of the same size as the corresponding study and specifies the probability of aneurysm for each · The HeadxNet algorithm was trained by Yeom, Allison Park, Standfrod graduate student in statistics and Christopher Chute, graduate student in computer science They outlined clinically significant aneurysms that had been detectable on 611 computerized tomography (CT) angiogram head scans The team labeled every voxel (the 3D equivalent of a pixel), indicating the




12 Best Travel Mosquito Nets For Camping In 21




Best Mosquito Net Hats And Mosquito Head Nets Insect Cop
· Artificial intelligence (AI) applications are growing at an unprecedented pace in health care, including disease diagnosis, triage or screening, risk analysis, surgical operations, and so forth Despite a great deal of research in the development and · New AI Tool, HeadXNet, to Help Detect Brain Aneurysms June 14th, 19 Siavash Parkhideh Informatics, Neurology, Neurosurgery, Radiology Researchers from Stanford University have developed a new AI · Using HeadXNet, clinicians correctly identified more aneurysm, reduced the "miss" rate and were more likely to agree with their colleagues Not only did the algorithm say whether the scan contained an aneurysm, but it also helped pinpoint the exact locations of the aneurysms "Search for an aneurysm is one of the most laborintensive and critical tasks radiologists can
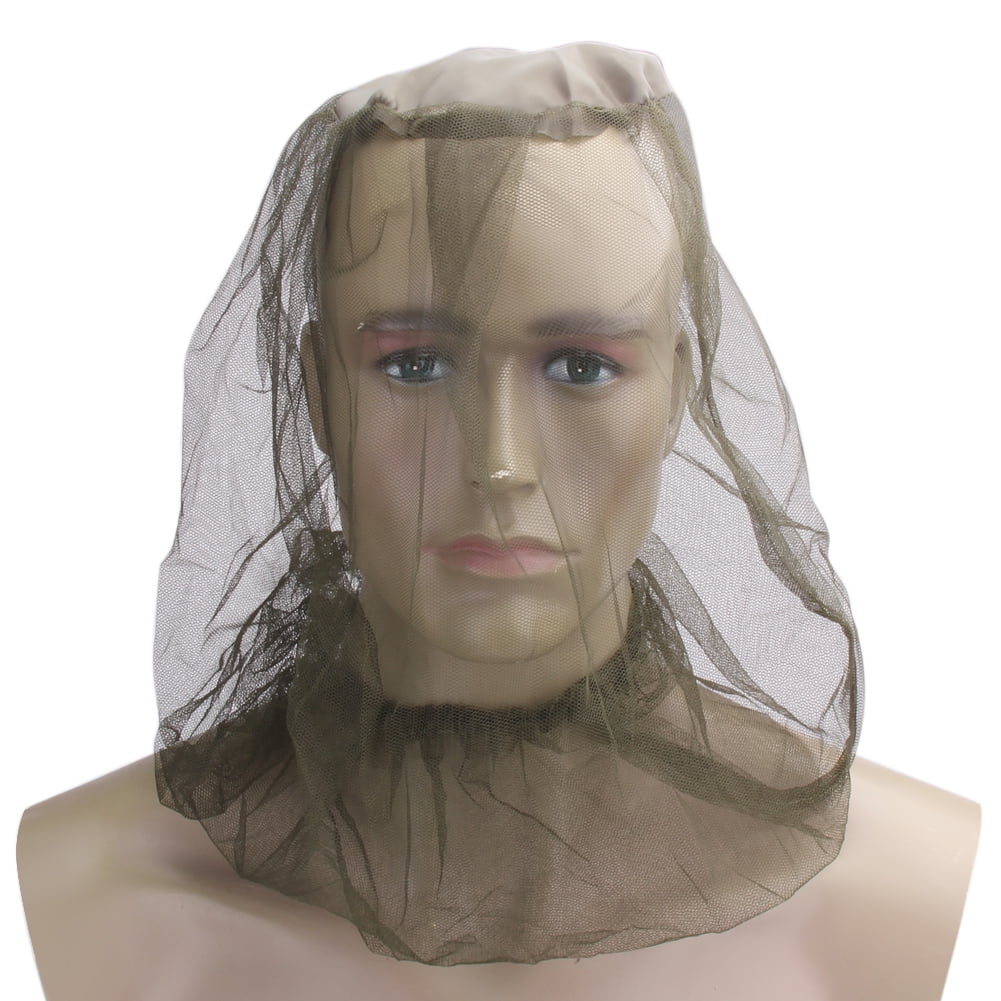



Aktudy Aktudy Midge Mosquito Insect Hat Bug Mesh Head Net Face Protector Travel Camping Walmart Com Walmart Com




Ben S Ultranet Head Net Ben S Tick Insect Repellent
Deep learning–assisted diagnosis of cerebral aneurysms using the HeadXNet model A Park, C Chute, P Rajpurkar, J Lou, RL Ball, K Shpanskaya, JAMA network open 2 (6), ee, 19 68 19 Fiber type composition and maximum shortening velocity of muscles crossing the human shoulder RC Srinivasan, MP Lungren, JE Langenderfer, RE Hughes Clinical Anatomy · Objective To develop and apply a neural network segmentation model (the HeadXNet model) capable of generating precise voxelbyvoxel predictions of intracranial aneurysms on head computed tomographic angiography (CTA) imaging to augment clinicians' intracranial aneurysm diagnostic performance · HeadXNet AI system helps radiologists detect brain aneurysms Doctors could soon get some help from an artificial intelligence tool when diagnosing brain aneurysms—bulges in blood vessels in the brain that can leak or burst open, potentially leading to stroke, brain damage or death
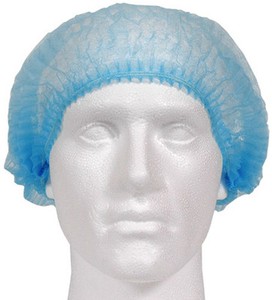



Mob Caps Head Cover Hair Net Various Colours And Quantities Food Industry Ebay
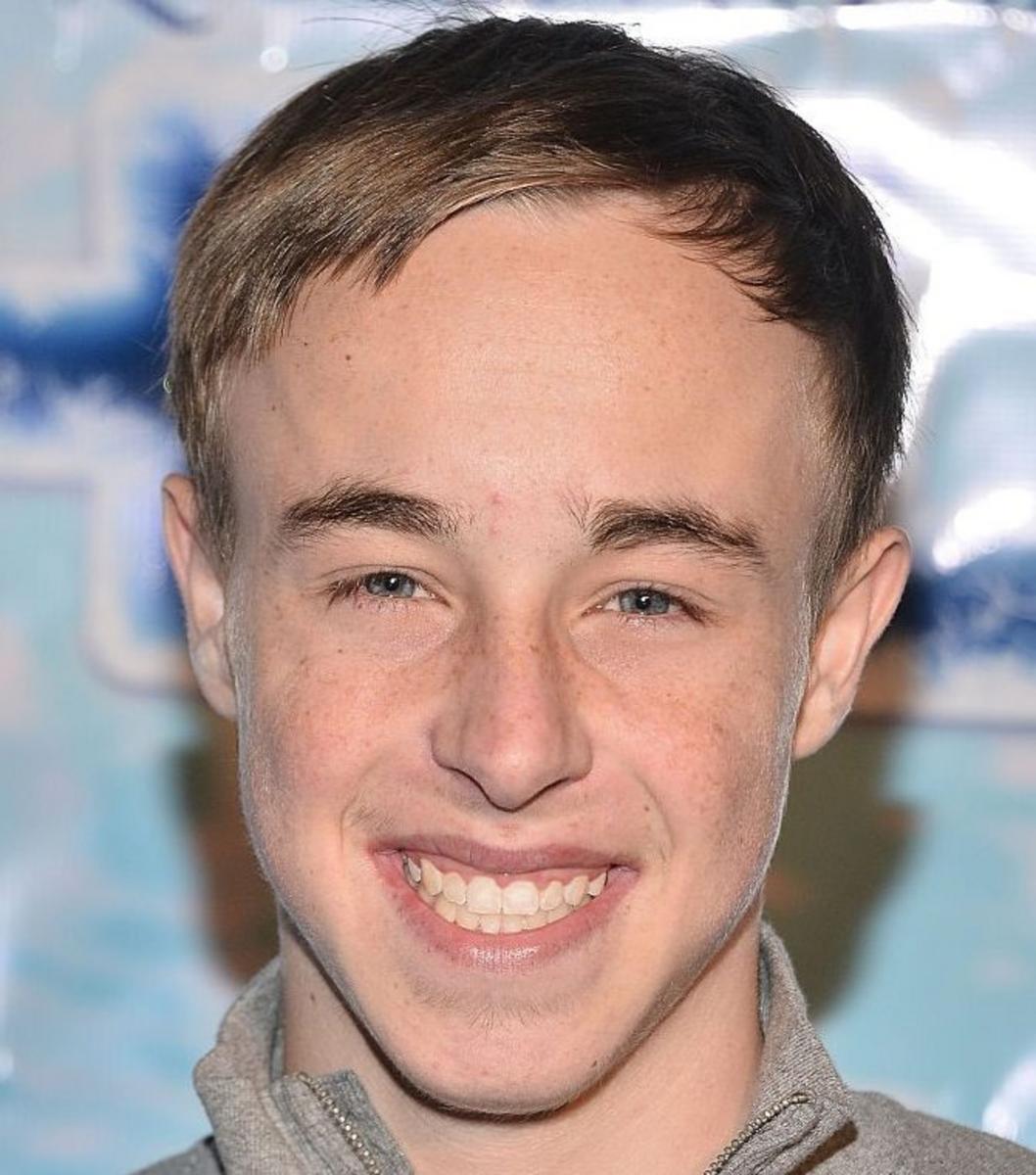



Jae Head Net Worth Celebrity Net Worth
· HeadXNet is a CNN with an encoderdecoder structure (eFigure 1 in the Supplement), where the encoder maps a volume to an abstract lowresolution encoding, and the decoder expands this encoding to a fullresolution segmentation volume The segmentation volume is of the same size as the corresponding study and specifies the probability of aneurysm for each voxel,The tool is built around an algorithm called HeadXNet and improved the ability of clinicians to correctly identify aneurysms at a level equivalent to finding six more aneurysms in 100 scans that contain them Image credit Allison Park AI to detect aneurysms 4 New research could help predict seizures before they happen Scientists at the Royal College of Surgeons in Ireland haveIn this study, we developed a 3dimensional (3D) CNN called HeadXNet for segmentation ofintracranial aneurysms from CT scans Neural networks are functions with parameters structured asa sequence of layers to learn different levels of abstraction Convolutional neural networks are a typeof neural network designed to process image data, and 3D CNNs are particularly well suited




Best Mosquito Head Net Reviews 17 Pandaneo




Best Mosquito Net Hats And Mosquito Head Nets Insect Cop
HeadXNet did not influence how long it took the clinicians to decide on a diagnosis or their ability to correctly identify scans without aneurysms – a guard against telling someone they have an aneurysm when they don't To other tasks and institutions The machine learning methods at the heart of HeadXNet could likely be trained to identify other diseases inside and outside the brain · The end result of the HeadXNet tool is the algorithm's conclusions overlaid as a semitransparent highlight on top of the scan This representation of theHeadXNet algorithm tool can detect brain aneurysms 7 June 21 Service Engineering Researchers at Stanford University have developed an AI tool, built on an algorithm called HeadXNet, which can identify parts of the brain that might contain an aneurysm The algorithm's conclusions are overlaid on computerised tomography angiogram head scans as a semi




Head Net For Clinic Rs 2 Piece Shree Bhavani Health Care Id
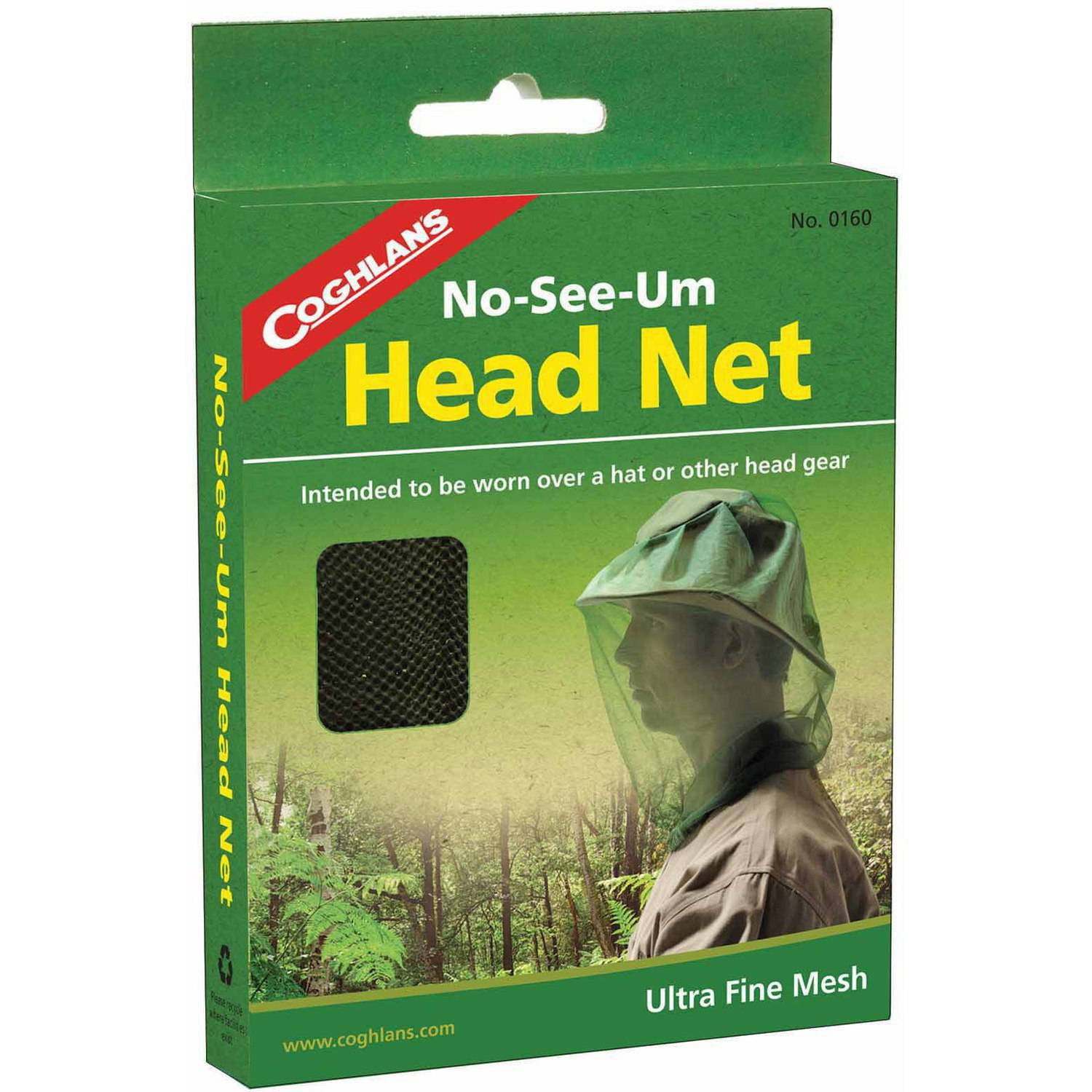



Coghlan S No See Um Head Net Walmart Com Walmart Com
Objective To develop and apply a neural network segmentation model (the HeadXNet model) capable of generating precise voxelbyvoxel predictions of intracranial aneurysms on head computed tomographic angiography (CTA) imaging to augment clinicians' intracranial aneurysm diagnostic performance · The end result of the HeadXNet tool is the algorithm's conclusions overlaid as a semitransparent highlight on top of the scan This representation of the · The study showed that in the detection of intracranial aneurysms, HeadXNet improved clinician specificity by about 15%, sensitivity by about 6%, and accuracy by about 4% While CTA is the primary imaging technique used to diagnose, monitor, and plan surgeries for intracranial aneurysms, analyzing the results is incredibly timeconsuming, even for the most




Sea To Summit Mosquito Head Net Gander Outdoors




Explore Headnets For Bugs Amazon Com
The researchers are also planning to establish a multicenter collaboration to make AI health care technologies, like HeadXNet, easier to use across different hospitals, which tend to have different hardware, patient populations and imaging protocols Given the challenges of making AI work in all these different situations and the vital importance of accurate diagnoses these researchers · Built around an algorithm called HeadXNet, the tool looks through head scans made using computed tomography angiography and for every voxel, the 3D version of a pixel, decides whether or not there is a sign of an aneurysm · HeadXNet was tested by eight clinicians by evaluating a set of 115 different brain scans for aneurysms, once with the help of HeadXNet and once without With the tool, the clinicians correctly identified more aneurysms, and therefore reduced the "miss" rate, and the clinicians were more likely to agree with one another The researchers believe that the tool did




Amazon Com Stansport Mosquito Head Net Sports Outdoors




Amazon Com Stansport Mosquito Head Net Sports Outdoors
· Deep learning helps detect intracranial aneurysms By Erik L Ridley, AuntMinniecom staff writer June 10, 19 A deeplearning algorithm called HeadXNet can improve the performance of clinicians of varied specialties and levels of experience for detecting intracranial aneurysms on head CT angiography (CTA) exams, according to research published online June 7 · Of these, 534 CT angiograms (6 aneurysms) were assigned to the training set, and the remaining 534 CT angiograms (649 aneurysms) constituted the validation set The sensitivity of the proposed algorithm for detecting cerebral aneurysms was 975% (633 of 649; · Based on an algorithm called HeadXNet, the new tool is designed to highlight the areas of a brain scan that could contain aneurysms, which have the potential to leak or burst open and could lead to a stroke, brain damage or death Researchers noted that the AI solution improved clinicians' ability to correctly detect aneurysms and also consensus among the interpreting



Sea To Summit Head Net Rei Co Op
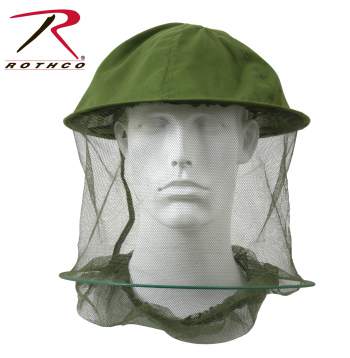



Mosquito Insect Netting
· The HeadXNet model was developed by a group of researchers at Stanford University, who trained the algorithm using brain scans from 662 patients Using this data, the model examines scans and · Nevertheless, HeadXNet serves as a significant step forward in the process of solving a complicated and fatal problem with underfunded research It also demonstrates the greater benefit of usingHeadXNet Archives Express Computer




The Best Mosquito Netting For Heads Hats Top 7 Tested



Mosquito Head Net Canadian Tire
· Deep LearningAssisted Diagnosis of Cerebral Aneurysms Using the HeadXNet Model Park A, Chute C, Rajpurkar P, Lou J, Ball RL, Shpanskaya K, Jabarkheel R, Kim LH, McKenna E, Tseng J, Ni J, Wishah F, Wittber F, Hong DS, Wilson TJ, Halabi S, Basu S, Patel BN, Lungren MP, · Eight clinicians tested HeadXNet by evaluating a set of 115 brain scans, once with the help of the AI and once without With the tool, the clinicians correctly identified more aneurysms and therefore reduced the "miss" rate The time it took to arrive at diagnosis was not affected Despite the early success, the Stanford team is cautious in relation to the clinical use of the toolThe end result of the HeadXNet tool is the algorithm's conclusions overlaid as a semitransparent highlight on top of the scan This representation of the algorithm's decision makes it easy for the clinicians to still see what the scans look like without HeadXNet's input "We were interested how these scans with AIadded overlays would improve the performance of clinicians," said




The Best Mosquito Netting For Heads Hats Top 7 Tested




Amazon Com Stansport Mosquito Head Net Sports Outdoors




Ben S Invisinet Head Net Ben S Tick Insect Repellent




Amazon Com Head Net




Amazon Com Head Net




Amazon Com Mosquito Head Net




Head Net Black Fly Mosquito




Amazon Com Coghlan S Mosquito Head Net Standard Insect Repellents Garden Outdoor




Top 5 Best Mosquito Head Nets 21 Review Pest Strategies




Ben S Invisinet Head Net Ben S Tick Insect Repellent




12 Best Travel Mosquito Nets For Camping In 21




Head Net Sea To Summit
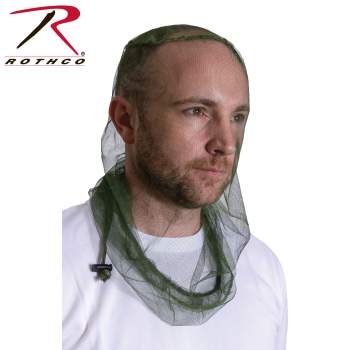



Mosquito Insect Netting




Amazon Com Sea To Summit Mosquito Head Net Mosquito Head Net Camping First Aid And Safety Equipment Sports Outdoors
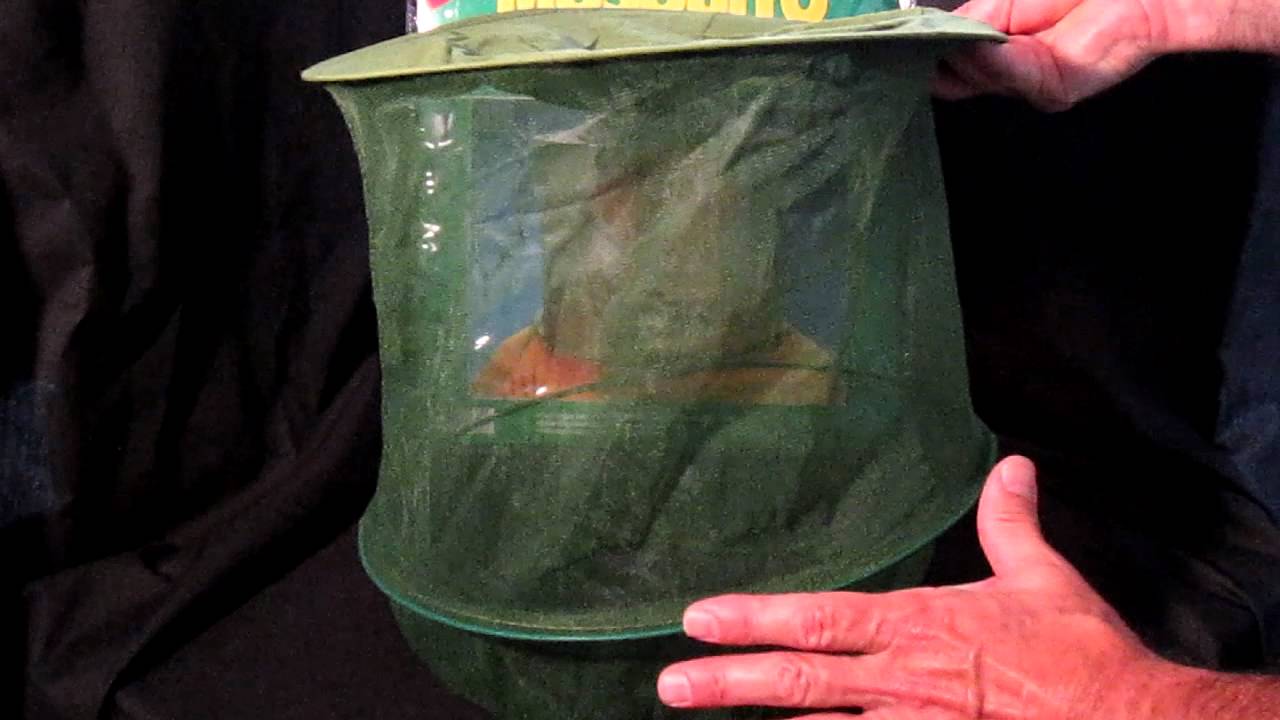



Coghlan S Mosquito Head Net Youtube




Amazon Com Head Net
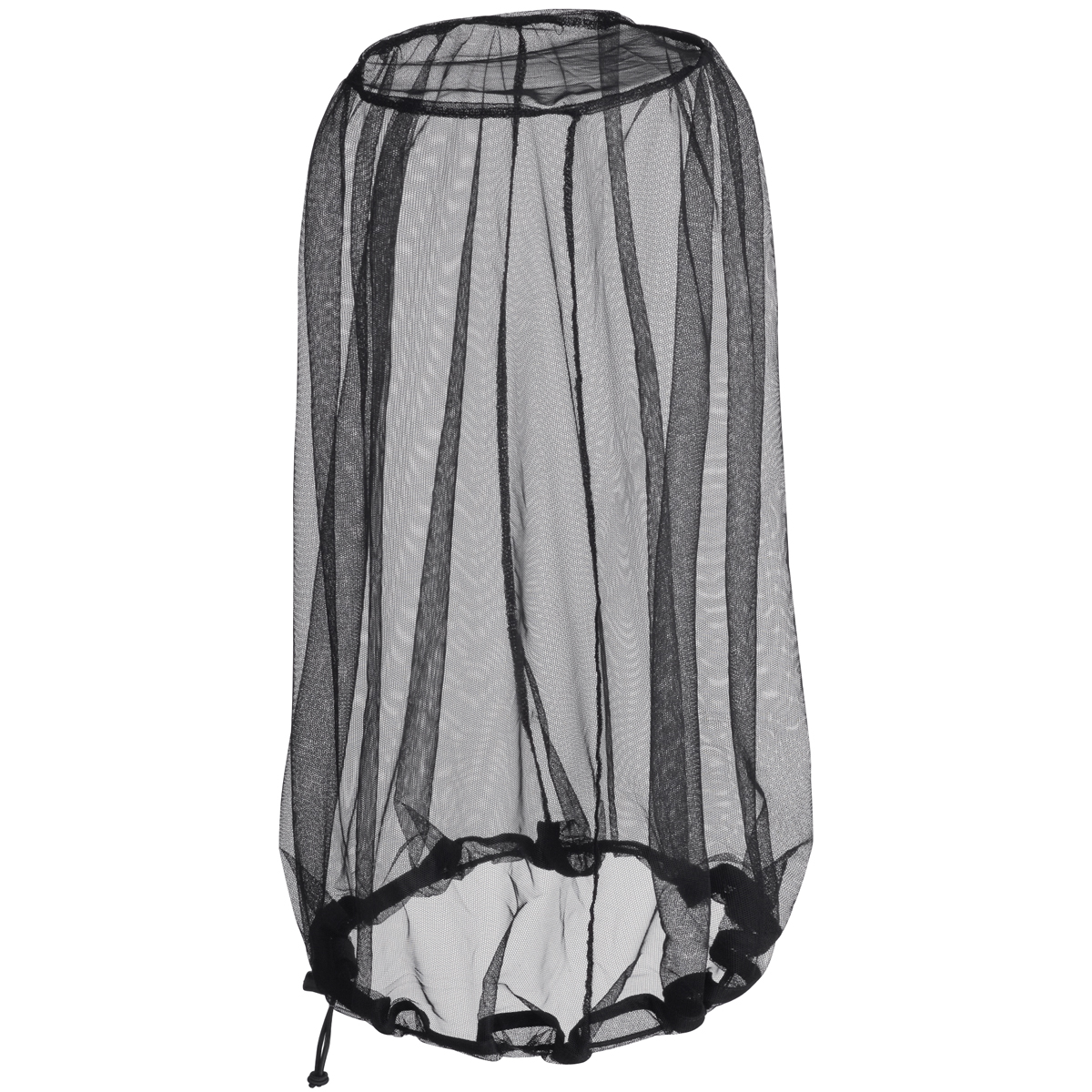



Onsight Bug Head Net Unisex Mec
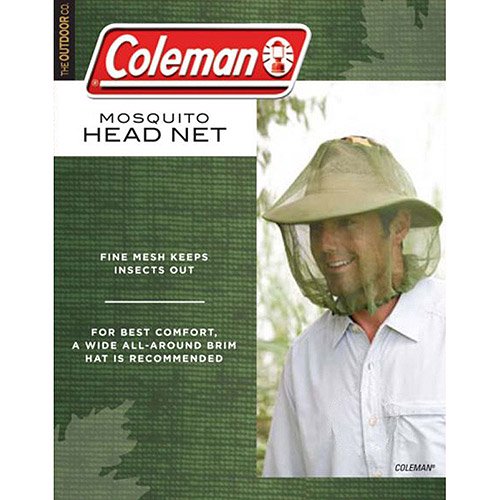



Coleman Mosquito Mesh Head Net With Brim Green Walmart Com Walmart Com
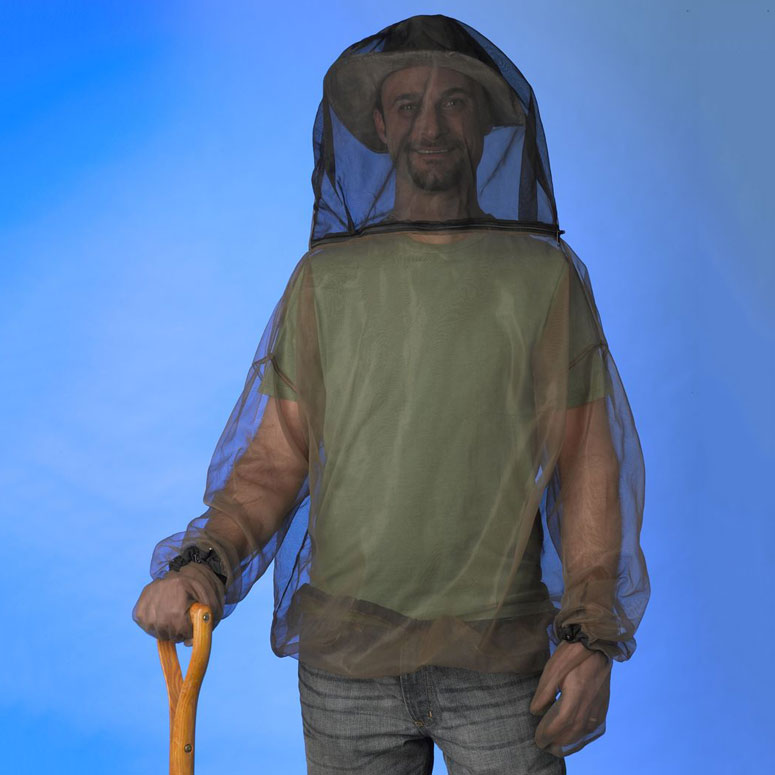



Bug Jacket With Head Net
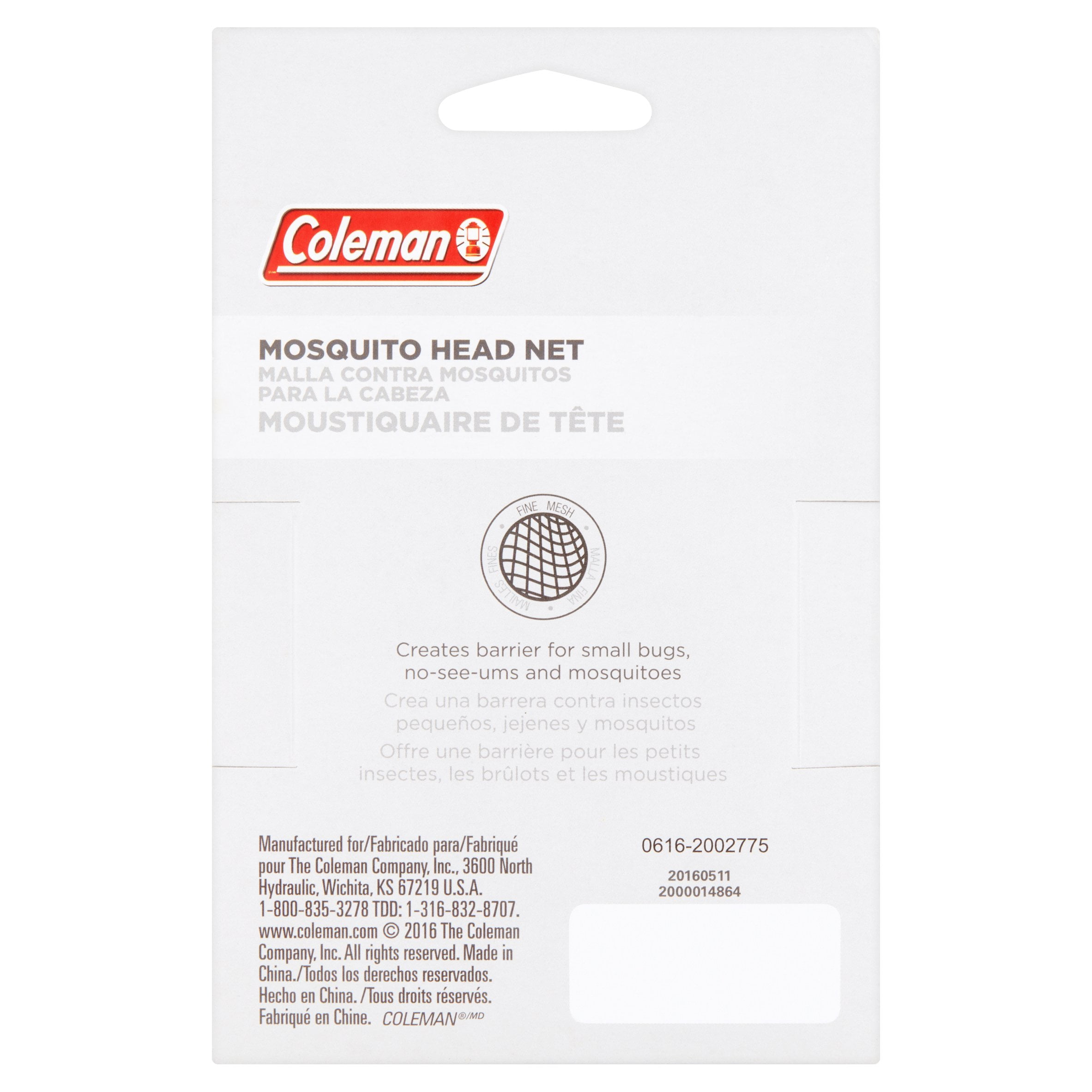



Coleman Mosquito Mesh Head Net With Brim Green Walmart Com Walmart Com




Sea To Summit Lightweight Ultra Mesh Head Net Sub Zero




The 18 Best Fishing Hats Of 21 Bucket Net Hats More




Amazon Com Bug Head Net




Amazon Com Mosquito Head Net




Amazon Com Ben S Ultranet High Visibility Head Net For Insect Protection Sports Outdoors




Best Mosquito Net Hats And Mosquito Head Nets Insect Cop




Amazon Com Even Naturals Premium Mosquito Head Net Mesh Ultra Large Extra Fine Holes Insect Netting Bug Face Shield Soft Durable Fly Screen Protection For No See Um Midges Gnats Carry Bag




Head Net Sea To Summit



World Famous Green Mosquito Head Net Home Hardware




Mob Cap Head Net B0601 Gloves Manufacturer Supplier Supply Head Protection Cap Bergamot Sdn Bhd




My Clothing System For Backpacking In Peak Mosquito Season Andrew Skurka




Amazon Com Coghlan S Compact Mosquito Head Net 7 9 X 19 7 X 43 3 Sports Outdoors




Amazon Com Luwint Mosquito Head Net Hat Breathable Sun Hat Beekeeper Hat Cap With Veil Clothing
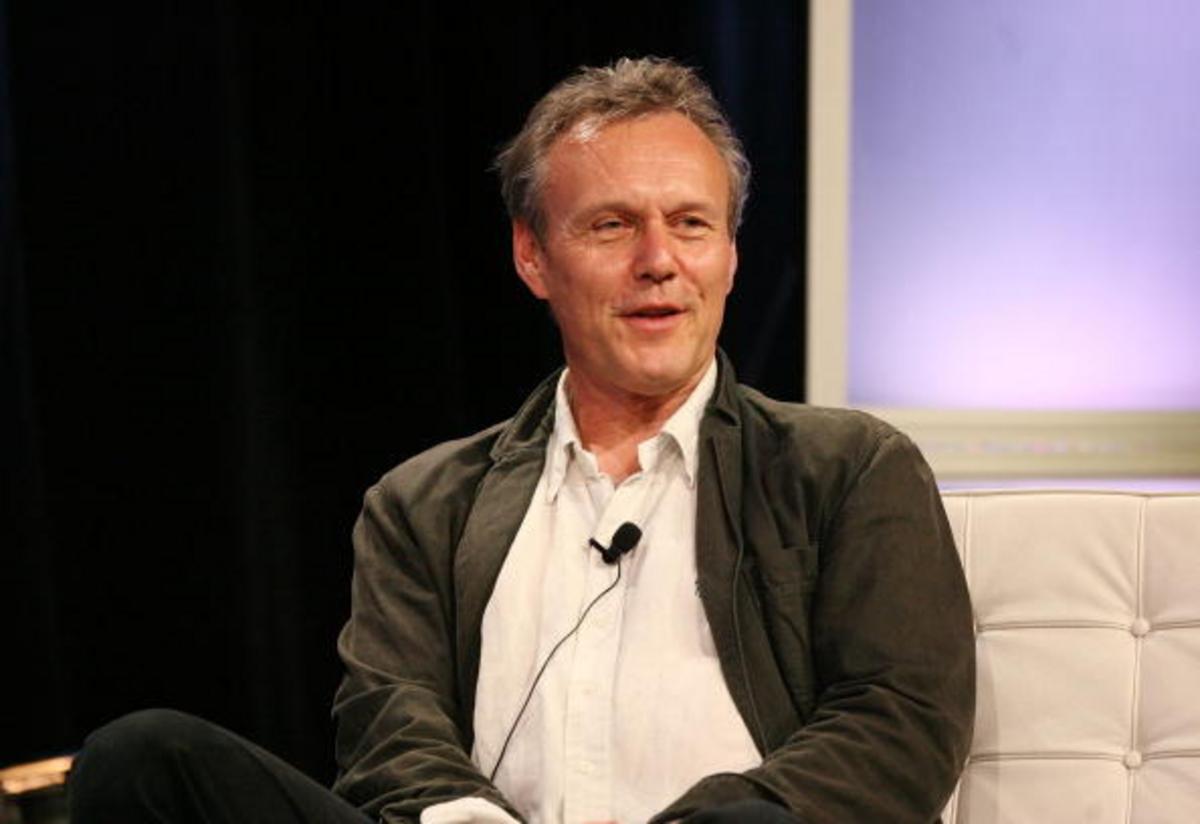



Anthony Head Net Worth Celebrity Net Worth




Amazon Com Coghlan S Mosquito Head Net Standard Insect Repellents Garden Outdoor
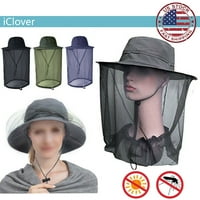



Mosquito Nets Walmart Com



Mosquito Net Clothing Rei Co Op



Ultralight Mosquito Headnet



Sea To Summit Mosquito Head Net With Insect Shield Rei Co Op




Amazon Com Outdoor Head Net Face Neck Protection Health Personal Care




Gross Head Net Head And Efficiency Of Turbine Mechanical Engineering Concepts And Principles




Head Net Disposable Head Cover Manufacturer From Ahmedabad



Field Stream No See Um Head Net Dick S Sporting Goods




Amazon Com Wemaker Head Net Premium Mesh Head Net Face Netting Soft Durable Lightweight Fly Screen Protection For Outdoor Fishing Hiking Beekeeping And Gardening Garden Outdoor




Mosquito Head Net Midge Head Net Lifesystems Latvia




Hunter S Specialties Nylon Net Head Net Realtree Xtra Camouflage Clothing Boots Wearable Gear Buy Online Guns Ship Free From Arnzen Arms Gun Store
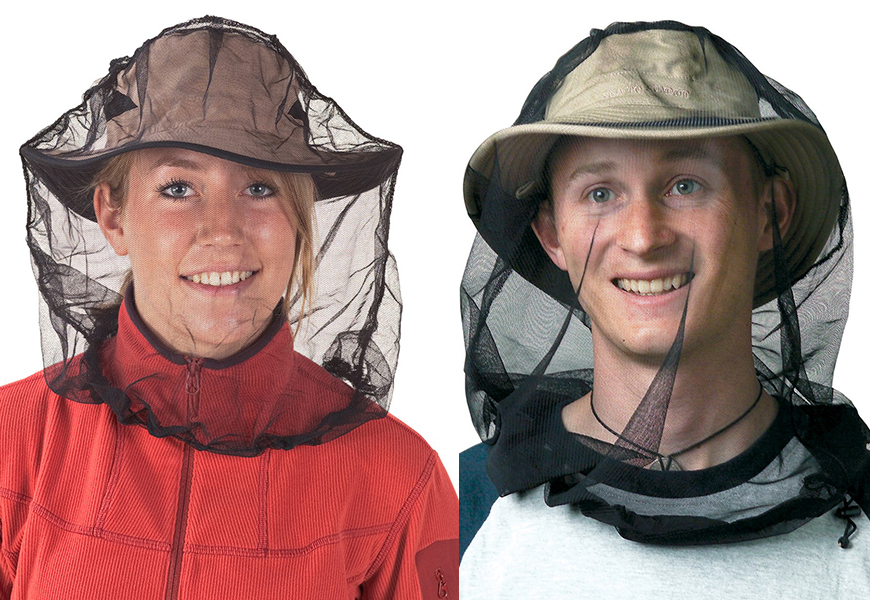



The Insect Repellent Clothing And Gear Every Traveler Should Have
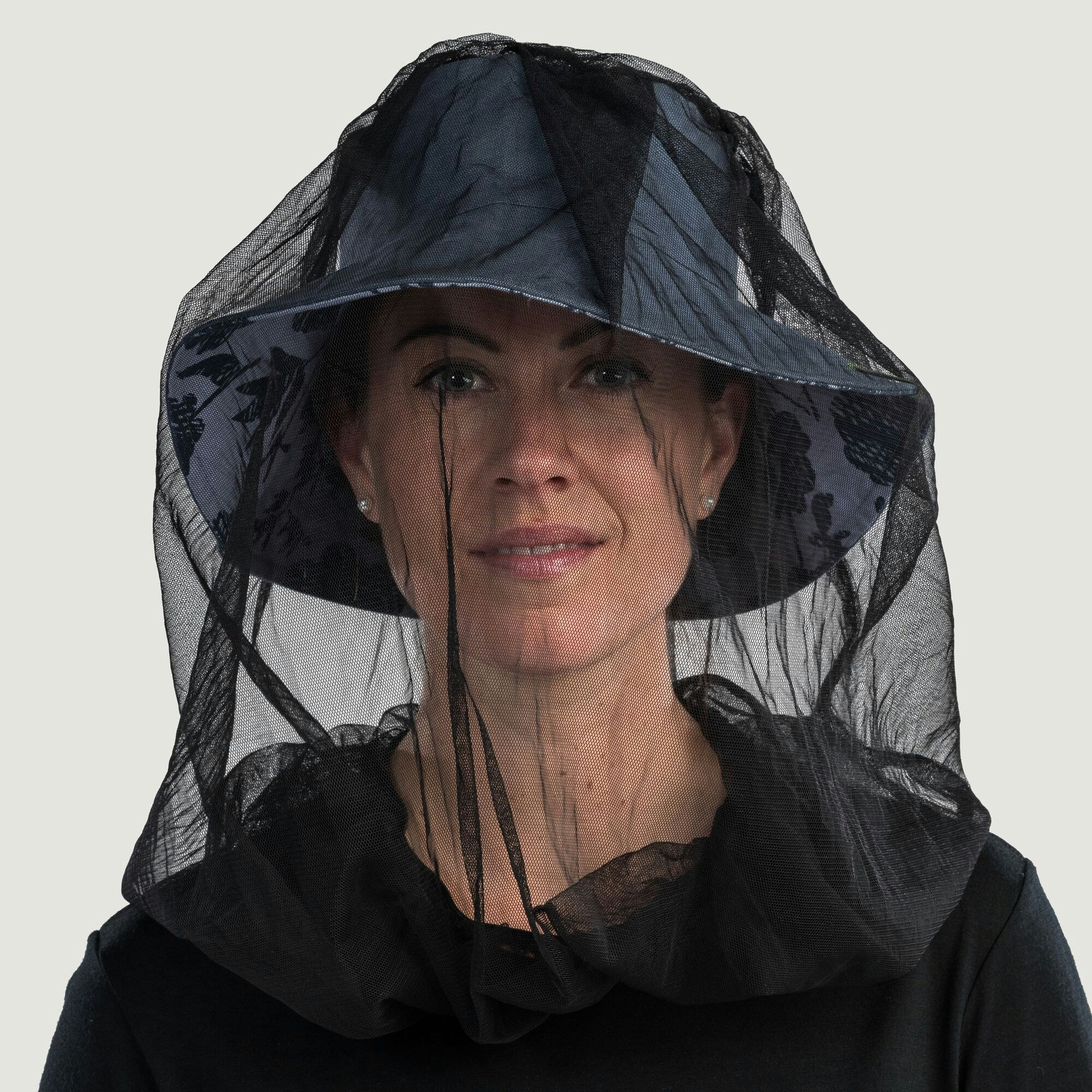



Mosquito Head Net




Trekmates Bush Hat With Mosquito Net Safariquip




Top 5 Best Mosquito Head Nets 21 Review Pest Strategies




Best Mosquito Net Hats And Mosquito Head Nets Insect Cop



Mosquito Insect Hat Bug Mesh Head Net Face Protector Travel Camping Hedging Anti Mosquito Cap Shopee Malaysia




Amazon Com Ben S Ultranet High Visibility Head Net For Insect Protection Sports Outdoors




Midges And How To Avoid Them Walking Advice From Walk Fife
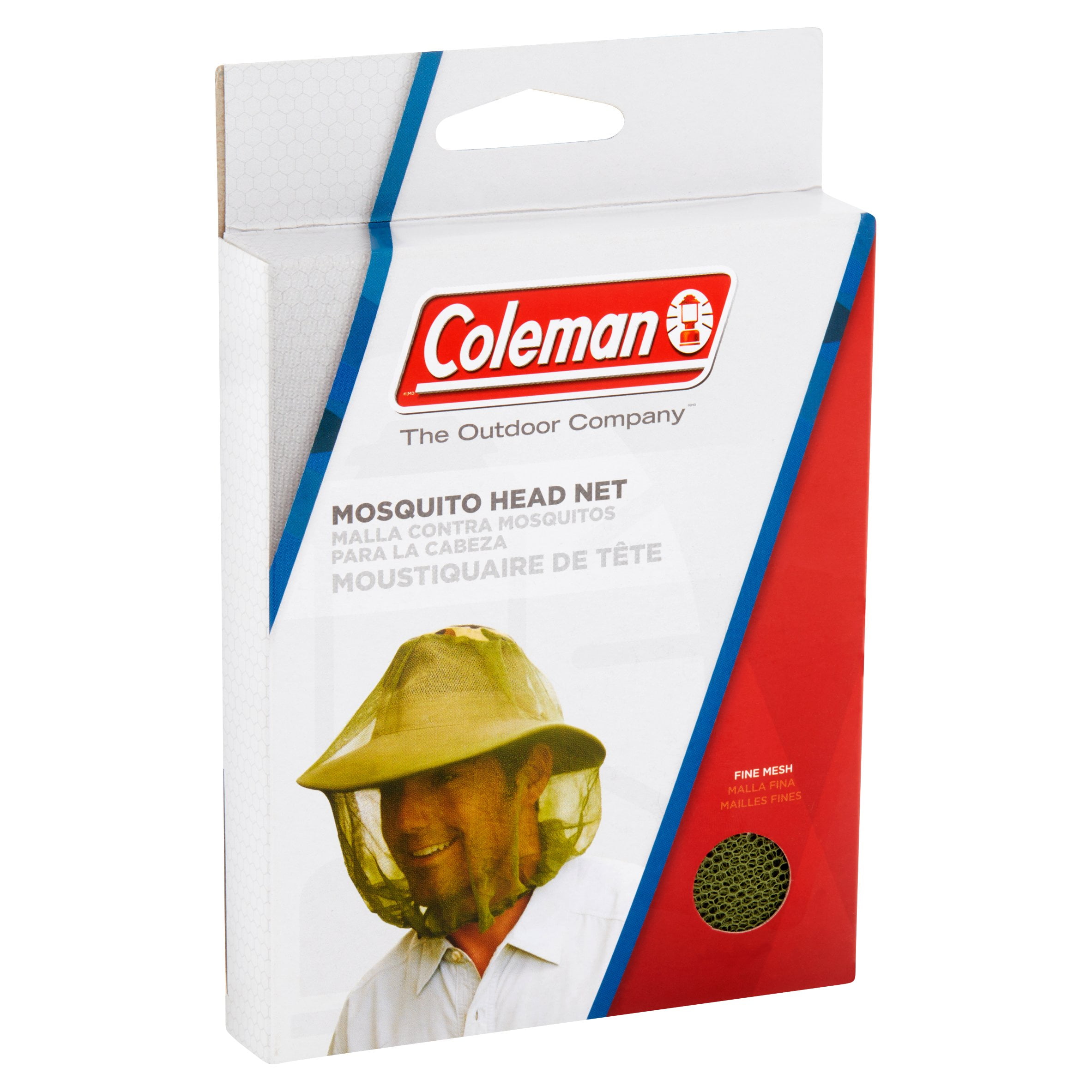



Coleman Mosquito Mesh Head Net With Brim Green Walmart Com Walmart Com




Insect Protective Headnet No See Um Mesh Bugbaffler Com




Lifesystems Midge And Mosquito Head Net Crib Netting




Amazon Com Head Net



Field Stream No See Um Head Net Dick S Sporting Goods




Best Mosquito Net Hats And Mosquito Head Nets Insect Cop




Amazon Com 4 Pack Head Net Face Netting Neck Cover Netting Mesh Net For Outdoor Activity Regular Size Grey Garden Outdoor




Ben S Invisinet Head Net Ben S Tick Insect Repellent




Amazon Com Head Net
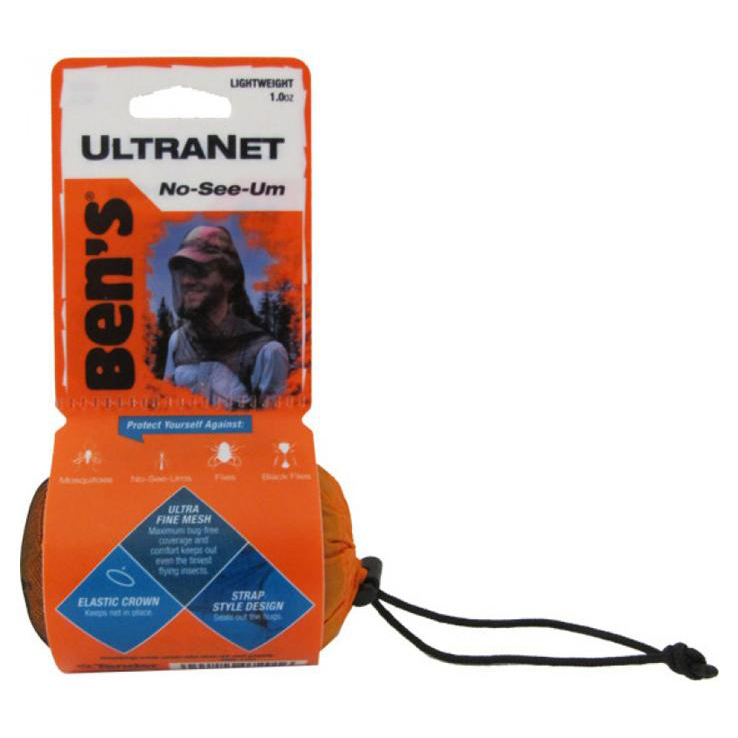



Ben S Ultranet Head Net Woodbine Moss Sportsman S Warehouse
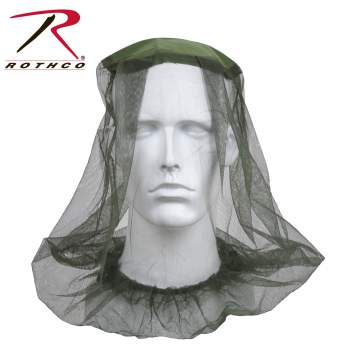



Mosquito Insect Netting




Jac O Net 137 146 156 157 255 Mirage Neutral Light Dark Medium Brown White Blonde Grey Black Hair Net With No Elastic Regular Bob Bouffant Size Triangle Hair Net Wave O




Amazon Com Head Net




Head Net Sea To Summit




Amazon Com Benvo Head Net Mesh Face Neck Netting From Insect Bug Bee Mosquito Gnats For Any Outdoor Lover With Free Carry Bags 2pcs White Updated Big Size Garden Outdoor




Amazon Com Wemaker Head Net Premium Mesh Head Net Face Netting Soft Durable Lightweight Fly Screen Protection For Outdoor Fishing Hiking Beekeeping And Gardening Garden Outdoor




Amazon Com Coghlan S Mosquito Head Net Standard Insect Repellents Garden Outdoor




Ben S Invisinet Head Net Ben S Tick Insect Repellent
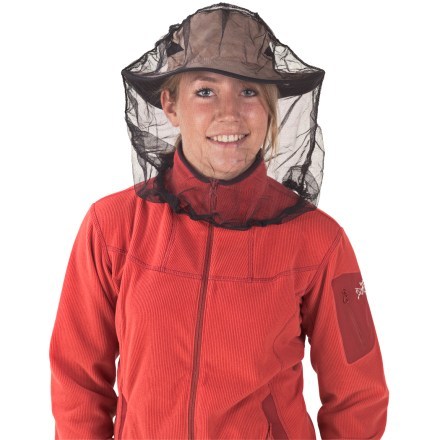



Sea To Summit Head Net With Insect Shield Gear Bug Protection
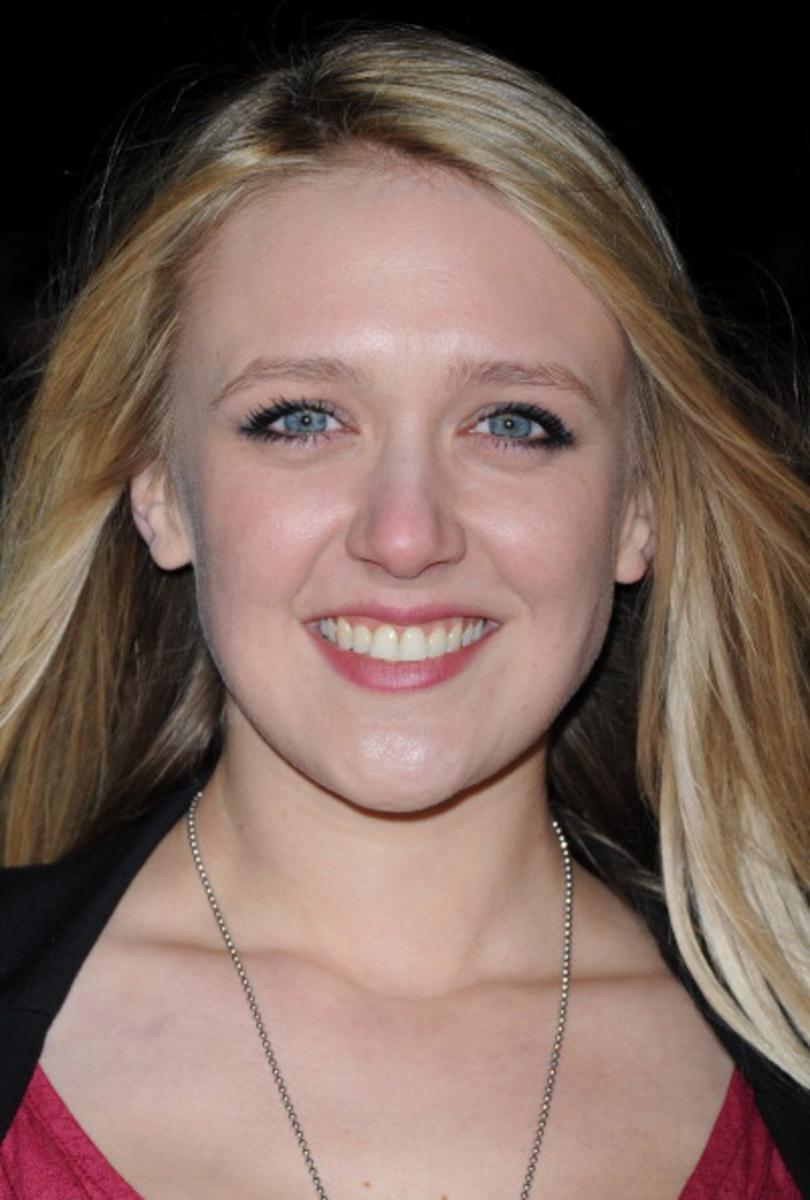



Emily Head Net Worth Celebrity Net Worth




Amazon Com Head Net



Ben S Invisinet Head Net Cabela S




Baby Lifesystems Midge And Mosquito Head Net Safety



Mosquito Net Clothing Rei Co Op
0 件のコメント:
コメントを投稿